AI NEWS. In the future, perhaps complications following subarachnoid hemorrhage can be reduced or the threat of an ensuing stroke can be avoided. A new monitoring system is being developed which will create an alarm and alert the physician if a patient develop signs of oxygen deficiency in the brain. In a joint project between medicine and engineering at the University of Gothenburg, researchers are using machine learning and AI to identify useful markers in the signals that are already collected today in neurology intensive care wards.
Helena Odenstedt Hergès is associate professor and adjunct senior lecturer in anesthesiology and intensive care at the University of Gothenburg. She is also senior physician at Sahlgrenska University Hospital Neurointensive Care Unit (Niva). The unit provides care and treatment for patients with serious cranial and spinal injuries and for acute neurological diseases.
Many of the patients at Niva, a few hundred per year, are admitted because of subaracnoid hemorrhage. This is a chategory of stroke that predominantly affects younger persons.
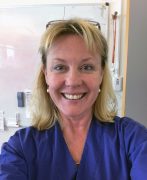
“When the patient is first admitted, the most acute measure is to secure the ruptured vessel to avoid rebleeding. About a week later there arises a significant risk that residual blood can lead to a chain of events, including cerebral vasospasm as well as activation of coagulation and inflammation together with increased oxygen demand in the brain, ultimately resulting in a stroke,” says Helena.
Signs that are difficult to detect
The staff at Niva can have difficulty identifying signs of incipient cerebral ischemia especially in patients being sedated or for those that have not yet fully regained consciousness. This is why Helena and her colleagues are studying whether it is possible to develop a new warning system based on non-invasive monitoring of available physiological signals that are already collected today. Their initial studies indicate that this would likely be possible. However, they also realized that they would be dependent on expertise in software development in order to utilize their large data files.
First medical application
Miroslaw Staron is a professor at the Department of Computer Science and Engineering, which is part of both Chalmers University of Technology and the University of Gothenburg. In his research, he develops software and often cooperates with architects, programmers, designers, and test leaders at large software development companies. The cooperation he has now begun with Helena is his first concerning an application in the field of medicine. He appreciates the opportunity to learn about the functions of the various signals that are monitored in an intensive care unit.
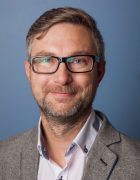
“It is quite exciting to be part of a team with researchers from so many different backgrounds, and I find it extremely interesting to learn about a new field. I am currently reading the literature to gain a deeper understanding of the various signals that are measured in intensive care and how we can use these signals to train AI,” says Miroslaw.
“It is impressive how much Miroslaw has learned about the field in such a short time. Much more than we clinicians have managed to learn about programming and artificial intelligence,” adds Helena.
Speaking the same language
The project uses machine learning, a type of artificial intelligence, to teach an algorithm to recognize reduced blood flow to the brain. Through analyzing large amounts of data, AI can discover patterns in real-time which otherwise would take a long time to analyze. Helena concludes that both she and Miroslaw are entering uncharted territory, but that their cooperation is working well.
“It is exciting to discuss issues with someone from an entirely different field. We have found a platform where we can speak the same language and, at the same time, increase our competency by gaining knowledge from another field,” says Helena. She explains that it takes a long time to develop new applications for the field of health care. There are absolute requirements for new methods to be both effective and safe.
“Before our research can result in a monitor that produces alarm signals when a patient’s condition has deteriorated, a lot of work needs to be put into evaluating the technique to ensure it functions adequately.”
Consideration also needs to be given to when it is appropriate to introduce AI-based systems in health care in light of ongoing digitalization. Within many fields, a great deal of development is required before the data to be analyzed by AI is available.
Operations as a research model
The research group has about ten members. Their cooperation has already led to one article and three more are in the process of being published in journals. So far, the results are based upon historical data from monitoring patients at risk for oxygen deficiency in the brain. The researchers are now studying signals in real-time using carotid surgery as their research model. This surgical procedure is used to treat stroke patients or those experiencing TIA (transient ischemic attack) and include temporarily reducing the blood flow to the brain which may cause brain ischemia mimicking the same pattern as in stroke patients.
“We are collecting synchronized information on heart rate variability, blood pressure, respiratory rate, electroencephalography and oxygenation, among other things. In contrast to the complications that can occur in patients with cerebral hemorrhage, using this model, we can monitor changes in the signals related to the onset of reduced blood flow to the brain. “
Ongoing recruitment to the study
Patients are currently being recruited to the study, and Miroslaw is continually adding new data to the algorithm.
“The clinical model is important if the algorithm is to be able to understand and identify the patterns that are relevant for further analysis. Through our cooperation, we have achieved n precision down to the minute of recognition of various events during the operation, as well as during post-operative care,” says Miroslaw.
He notes that they need data from different types of patients to develop a system which is robust, cost effective, and user friendly.
“An important part of the study is to see that we do not have too many false positive results, as that indicates a risk that the system could be unreliable. We also need to optimize the number of signals used to develop a reliable system that others will also be able to use.”
BY: ELIN LINDSTRÖM